Case Study
Newsletter marketing with personalized product recommendations for MediaMarkt
How the newsletter conversion rate can be increased by 500% through personalization with artificial intelligence.
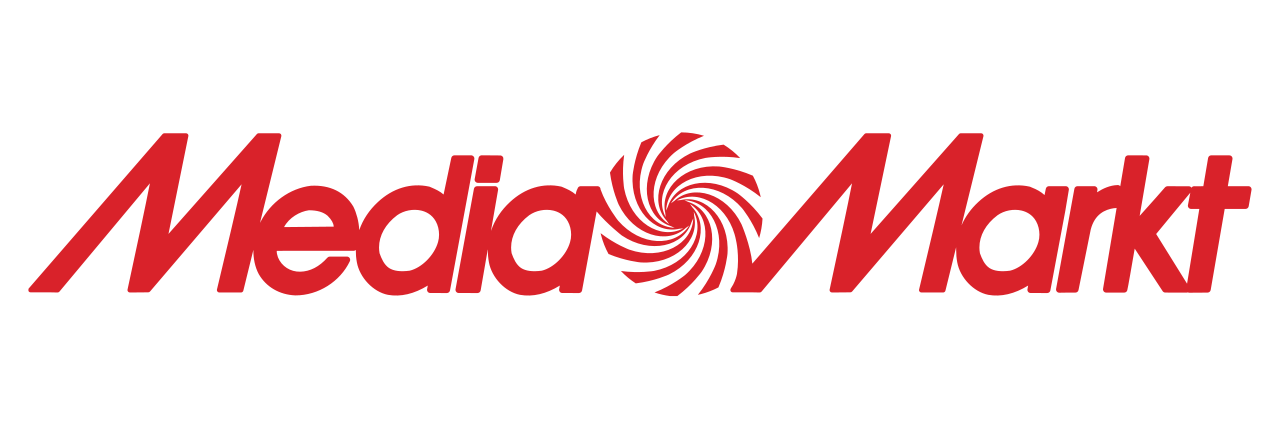
A newsletter is sent to MediaMarkt Club members every month. The performance of the newsletter in German-speaking countries is to be optimized. The performance is measured in: Sales per contact, conversion rate (i.e., click-to-buy rate), click-to-open rate.
7LYTIX should deliver four personalized product recommendations per customer or club member, using artificial intelligence. The performance of the product recommendations from 7LYTIX should then be compared with the product recommendations that were displayed by MediaMarkt itself.
We received the following data from the MediaMarkt Customer Analytics team as a basis for personalized product recommendations: sales data, anonymized demographic customer data, product data and historical newsletter data.
The latest methods of artificial intelligence were used, especially deep neural networks, which are able to represent customer-product interactions. And as the timing of offers also plays a decisive role, the models have also learned to rank products depending on the time of the offer.
7LYTIX provided a list of 4 personalized product recommendations for more than one million club customers. Then it was evaluated whether these customers had bought the recommended products in the 7 days after receiving the newsletter. And the results after two runs were quite interesting:
- A/B test: mailing end of 2019
- KPI: conversion rate in each group
- Result: more than 500 % Uplift for 7LYTIX at product level (e.g., a specific TV) and 6,5 % at product group level (other TVs). For the sake of completeness, it should be mentioned that MediaMarkt achieved a higher total shopping cart value with its own recommendations.
7LYTIX recommended far fewer products than MediaMarkt (300 vs. more than 8,000). The algorithm or our deep learning model learned to achieve a higher conversion rate.
- A/B test: mailing beginning of 2020
- KPI: Ratio of the conversion rate to the Holdout Group - i.e., which recommended products were purchased compared to a test group that did not receive any product recommendations.
- Result: +53,0 % for 7LYTIX, +21,5 % for Media Markt in comparions to the Holdout group.
You must carefully define what you want to optimize. Changing the objective has a big impact on the results, so you should be aware of the business value of individual metrics. The bottom line is: 7LYTIX achieved better results than MediaMarkt itself.
We have shown that our Deep Neural Networks approach is very flexible when it comes to 'learning' how to optimize various targets or KPIs for a given task. It has also been confirmed that it is essential to precisely define the setup and what is to be optimized. The Senior Manager for Innovation Projects at MediaMarkt, Dr. Christopher Bayer confirmed that the cooperation with 7LYTIX resulted in a very steep learning curve for both - for MediaMarkt and, thanks to the great cooperation, also for 7LYTIX.